GenAI chatbots struggle with factual precision
Generative AI chatbots, despite their flashy capabilities, stumble on what should be the easiest task: delivering accurate answers to straightforward questions. OpenAI’s SimpleQA benchmark drives this point home. Imagine asking a chatbot, “Who painted the Mona Lisa?” or “What year did the Titanic sink?” Simple stuff, right? For humans, yes. For these models, not so much. SimpleQA was built to test factual accuracy in a rigorous, repeatable way, and the results are sobering.
OpenAI’s own o1-preview model scored just 42.7% accuracy on this test. GPT-4o wasn’t far behind, with 38.2%. The smaller GPT-4o-mini plummeted to a dismal 8.6%. And it wasn’t just OpenAI struggling. Anthropic’s Claude-3.5-sonnet performed even worse, managing a mere 28.9%. Grade-wise? They all earned an F.
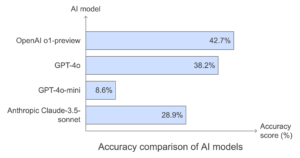
Accuracy comparison of AI models
These failures don’t just stem from lack of knowledge. The issue is deeper: hallucinations—confidently generating incorrect answers—and a troubling overconfidence in their outputs. Not only are these chatbots wrong, they’re wrong with conviction, and their mistakes can be hard to spot.
The benchmark itself forces these models into a tough spot. SimpleQA asks questions that demand precise, indisputable answers—no fluff, no hedging. In real-world use, these gaps in factual reliability could have serious implications, whether it’s in education, customer service, or any field relying on these tools for quick, factual responses. For now, they remain impressively flawed, great at sounding smart, but often falling short of simply being accurate.
Chatbots perform better with context and open-ended prompts
Chatbots are like storytellers: give them a canvas, and they’ll paint a picture. Hand them a blank sheet with no instructions, and they’ll struggle. The SimpleQA benchmark exploited this Achilles’ heel by stripping away all context, asking self-contained, precise questions. This format left the chatbots struggling to pin down exact answers.
Large Language Models (LLMs) do very well on open-ended prompts. They excel when tasked with generating nuanced summaries, exploring abstract ideas, or discussing complex topics. Ask them to draft a report on renewable energy or summarize a novel, and they’re in their element. But ask them, “What is the capital of France?” and they might give a clear answer—or flub it, depending on how the question is phrased.
The SimpleQA test exposed this gap. It penalized minor variations in responses, like hedging or offering alternative possibilities. The models simply weren’t built for the rigid, black-and-white precision the test required. Think of them as conversational multitaskers, not single-answer specialists.
The lesson here is simple but powerful: if you want your chatbot to perform well, feed it context. A few lines of background can make all the difference. The models might struggle with one-liners, but their ability to weave ideas into meaningful, context-rich responses is where their real strength lies. Use them for depth, not trivia, and you’ll see better results.
LLMs lack real-world understanding and structured knowledge
AI models are like encyclopedias that can talk—impressive, but limited. Research from MIT, Harvard, and Cornell reveals a deeper flaw: these models don’t “know” the world in any meaningful way. They generate answers based on patterns in their training data, not on an internal map of how things actually work.
Here’s an example. LLMs can give excellent driving directions through New York City when everything’s running smoothly. But when researchers closed just 1% of the city’s streets, the accuracy of these directions dropped from nearly 100% to 67%. Why? Unlike humans, these models don’t visualize the city or adapt to changes. They don’t “see” a detour, they just know what similar scenarios looked like in the past.
This isn’t a navigational issue. It reflects a broader inability to think flexibly or hold structured knowledge. Humans can improvise when the unexpected happens. AI can’t. Its brilliance in controlled tasks crumbles when thrown into diverse, unpredictable situations.
“Treat LLMs as tools, not thinkers. They’re powerful for automating repetitive tasks or processing large datasets but aren’t ready to replace human intuition or real-world decision-making. AI can imitate expertise, but it can’t replicate understanding—not yet.”
AI hallucinations pose real-world risks in critical industries
When AI hallucinates, it’s not harmless. In industries like healthcare, the consequences can be dire. Tools like OpenAI’s Whisper are widely used, especially for medical transcription. Over 30,000 clinicians and 40 health systems rely on Whisper-based solutions, such as Nabla, which has handled an estimated seven million medical visits in the US and France. But here’s the problem: these tools frequently fabricate information.
One analysis found hallucinations in nearly 40% of transcripts reviewed, and many were labeled harmful or concerning. Whisper even invented a non-existent medication, “hyperactivated antibiotics.” Imagine the risks—misdiagnoses, improper treatments, or worse—if such errors go unchecked.
The scale of adoption is staggering. Whisper has been downloaded 4.2 million times from HuggingFace, an open-source platform. While millions benefit from faster, more efficient workflows, the technology’s flaws are being embedded into critical processes without sufficient safeguards.
For leaders, the takeaway is clear: proceed with caution. AI-driven tools like Whisper are great for reducing workload and improving efficiency, but their outputs must be carefully validated. Build redundancy into workflows and always involve human oversight when the stakes are high.
Users should approach AI output with skepticism and verify information
Chatbots are great companions for brainstorming, summarizing, and ideation. But when it comes to delivering hard facts, they’re unreliable. Every output should be treated as a starting point, not gospel truth. This is especially important for professionals using AI in high-stakes settings.
Verification is key. Don’t just take an AI’s word for it; cross-check against original documents or reputable sources. Tools like Google’s NotebookLM make this process easier. For example, you can upload a research paper into NotebookLM, then compare its insights with what your chatbot provided. It’s a fast way to spot errors or inconsistencies.
Here’s the golden rule: never copy-paste AI responses into something important without scrutiny. AI-generated language often feels slightly off, whether it’s the phrasing or the emphasis. And worst of all, the bot might be hallucinating, confidently offering information that’s flat-out wrong.
For executives, this boils down to a mindset shift. AI isn’t here to replace critical thinking. It’s a tool to amplify it. Use it to speed up research, explore ideas, or lighten your workload, but always keep your own expertise in the driver’s seat. When you pair human judgment with AI’s capabilities, that’s when things get interesting.