Generative AI promises big, but most projects underdeliver
Generative AI is creating a storm of enthusiasm. Executives everywhere want in, hoping to be the first to leverage AI’s capabilities. Sadly, this is where it often falls apart: Gartner reports that 85% of these AI projects are stalling or flat-out failing to meet expectations. That’s a brutal statistic for any enterprise leader to stomach.
Imagine starting with big dreams of transformative AI, only to find the project hits roadblock after roadblock, or worse—gets abandoned entirely. Most enterprises jump in without a clear vision, expecting results that their current systems, data, and structures simply can’t support. Projects kick off with sky-high expectations but are quickly stifled by challenges that weren’t foreseen or strategically prepared for.
Why do they fail then? Well, because building generative AI solutions isn’t only about the tech. Instead, focus should be more on deeply aligning this tech with your data, business goals, and processes, from day one.
Too many projects chase trends without assessing what their organization can realistically support. Enterprises keep repeating this cycle, burning through resources without the outcomes they banked on. It’s the classic tale of trying to fly before learning to walk.
Cloud infrastructure demand surges with AI, but success remains limited
Generative AI has set off a frenzy for cloud infrastructure. Enterprises are stacking up on cloud storage and computational resources like never before, driven by AI’s high data and processing demands. Cloud providers are thrilled (of course!)—more resources mean more revenue. Here’s where it gets tricky though: investing in all that infrastructure doesn’t necessarily mean your AI project is going to take off. There’s a gap, a disconnect between the spend and the outcomes.
This isn’t the first time companies have thrown money at a problem, hoping tech alone would be the answer. We saw this happen with ERP systems, and we’re seeing it again here. Enterprises are pouring cash into the cloud, expecting it to magically overcome every AI hurdle.
Without a rock-solid strategy on how to make that cloud investment actually pay off, most projects are bound to disappoint.
You can have the best tools, the fastest processors, but without a blueprint, the results won’t follow. Cloud resources are just a vehicle. If you don’t know where you’re going, the car’s horsepower won’t make a difference.
Data quality issues are a core reason for AI project failures
The dirty secret of AI is this: it’s only as good as the data you feed it. Generative AI demands top-notch, accurate data, but for many enterprises, data quality is a real Achilles’ heel. Years of neglect, scattered data sources, and tech debt have left most companies struggling with poor data hygiene. And to get AI up and running, this neglected data needs to be fixed. Fast. But cleaning up that mess is neither cheap nor easy.
Fixing data problems is not cleaning up a spreadsheet, let’s be clear on this. The entire foundation needs to be rebuilt.
Many enterprises have relied heavily on legacy ERP systems or jumped into hyped trends like data warehouses, which haven’t aged well. Now they’re sitting on mountains of inconsistent or irrelevant data, and they’re finding out that AI won’t work unless they address these issues head-on. It’s a massive challenge, and for some leaders, it’s a career risk. One CIO put it bluntly (no, we’re not naming names): “I’m not going to take a hit for somebody else’s bad decision.”
Hesitation creates a huge obstacle. Leaders know their data isn’t in great shape, but they’re often reluctant to shoulder the cost and political fallout of addressing it. And so, projects stall, data remains uncleaned, and AI potential sits untapped.
Growing divide between AI “Haves” and “Have-Nots”
As generative AI adoption grows, we’re seeing a clear divide between the “haves” and “have-nots.” Companies that manage their data well are quickly gaining an edge, turning their AI projects into powerful, differentiating assets. Those without high-quality data, though, are missing out—unable to make AI a functional part of their business strategy.
The divide keeps growing. If your company invests in solid data strategies, AI can become a tool for real competitive advantage. For those who don’t? They’re left struggling, watching competitors leverage AI to move ahead. It’s a choice: you either get serious about data management, or you fall behind. As AI continues evolving, that gap between those with well-oiled data engines and those without will only widen. For companies that lag now, catching up won’t be easy.
Cloud infrastructure alone won’t guarantee AI success
Here’s where many companies go wrong: they think pouring money into cloud infrastructure will somehow guarantee AI success. It doesn’t work that way. While cloud infrastructure provides the resources, real success with AI depends on far more than just storage and compute power. It needs governance, careful planning, and the right talent to make it work. Enterprises without these key elements are bound to find themselves frustrated, with lots of cloud spend but little to show for it.
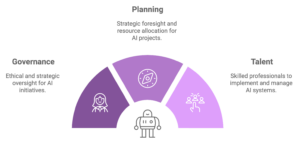
What’s required for success with Generative AI?
Many companies are running head-first into the cloud without the underlying strategy. The result? A market correction might be in the cards as enterprises realize they need more than infrastructure—they need vision. Without these foundational pieces, the cloud can’t carry AI projects over the finish line. AI success doesn’t come from the cloud alone. Rather, it comes from strategic thinking that aligns resources with results.
Leadership courage is key for tackling AI challenges head-on
Generative AI isn’t for the faint-hearted. Implementing it successfully requires a hard look at core business issues, especially around data quality and strategic direction. It takes leaders willing to make tough calls, often politically risky, to get it right. And that’s where many struggle—CIOs and executives know that fixing these foundational issues will require serious commitment and investment.
Leaders with the courage to address these obstacles head-on can transform AI into a powerful business tool. But without that courage, they, and their organizations, are stuck. They keep running AI projects that look good on paper but fail to deliver.
To make AI truly work, leaders need to put in the time, energy, and resources—and, yes, take a few risks along the way.
Final thoughts
So here’s the question you have to ask yourself: are you just fueling the cloud provider’s bottom line, or are you truly positioning your brand to succeed with generative AI? It’s easy to throw money at infrastructure, and completely miss the real challenge of finding and using the right data, the right strategy, and the courage to address core issues head-on.
If your AI initiatives keep stalling, maybe it’s time to look in the mirror and decide if your team is equipped to adapt—or if you’re going to let your company fall behind. Are you ready to make the tough calls that will set your brand apart, or are you content watching others take the lead?